The secret behind Big Data-Data Science
When scrolling through your favorite social media app, liking memes, and sharing funny videos, have you ever wondered how the order of the posts on your feed was determined? What marketing content should the company use to attract more people to their app? Or have you pondered what contributes to trendy videos on the internet? To answer these questions, data science comes into play. Data science utilizes a combination of machine learning, statistics, and research methods to make sense of the vast amount of information at hand. It reveals valuable insights in the data to support processes for building the functions mentioned above.
BY Shao-Fang (Pam) Wang
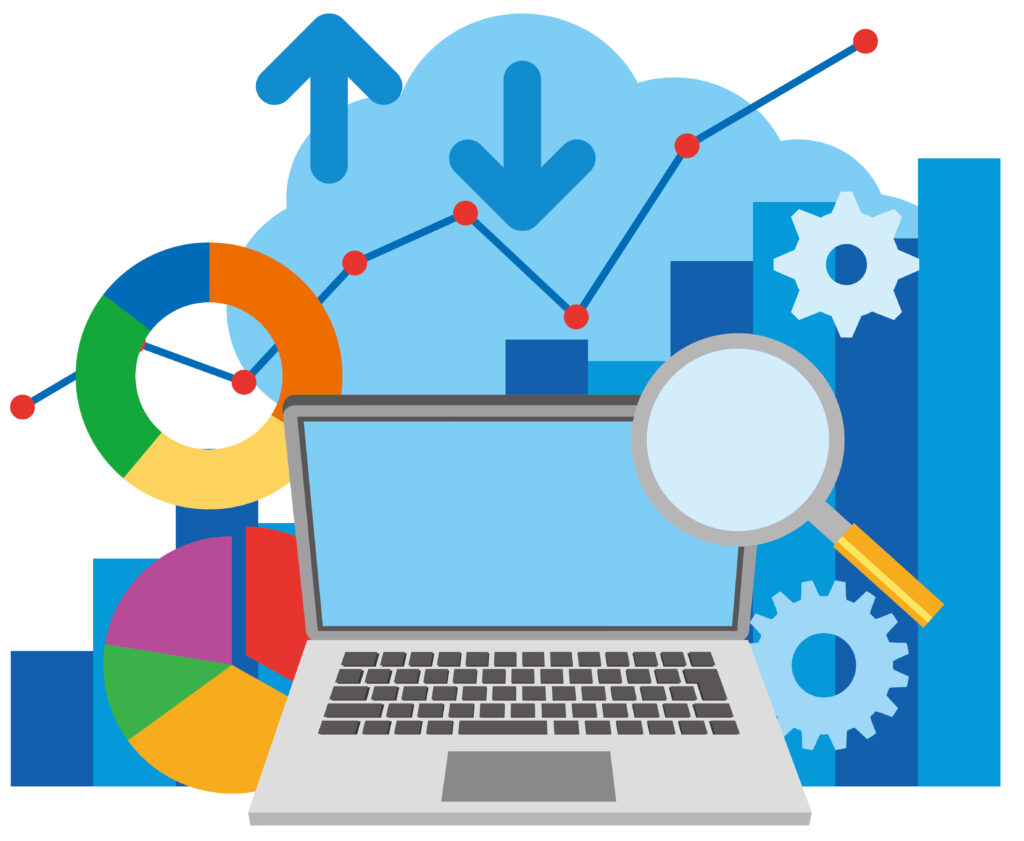
What is Data Science?
In essence, data science is the practice of applying advanced analytics to extract insights from datasets and translate that knowledge into actionable strategies. For example, by analyzing user data on a social media platform, we can understand what influences users' preferences for "liking" posts. Some users "like" the first few posts they see, regardless of the topic, while others prioritize "liking" posts that align closely with their interests regardless of the placement. Furthermore, users cannot “like” content they haven't viewed or that hasn't been shown to them. This comprehension empowers us to improve the presentation of posts on the feed and ultimately enhance the satisfaction and usage of the app for its users.
In addition to social media, data science permeates every other aspect of our lives, influencing fields such as e-commerce, medicine, finance, and sports. In medicine, for instance, researchers leverage data science to predict the likelihood of future cancer probability by analyzing patients’ imaging data. In sports, teams can utilize athletes' performance and health data to predict the athlete's future health and devise the training regimen most likely to keep them healthy until the finals. Similarly, businesses harness the power of data science to predict market trends, optimize promotional strategies, and prevent losses due to fraud. For example, an e-commerce website may use payment transaction data to identify fraudulent sellers or buyers, preventing financial losses and enhancing the user experience.
The data science process typically begins with a business problem or a research question, setting the stage for a systematic approach to harnessing valuable insights from data. Let’s say we want to improve usage of Instagram. Imagine that, based on previous research, we have discovered a positive correlation between the frequency of using the "like" button and the user's overall engagement with the app. More specifically, users who frequently engage with content by clicking the 'like' button tend to revisit the app more often and spend longer durations on it. Therefore, understanding users' behavioral patterns of clicking the “like” button could help identify strategies to enhance Instagram products and foster increased app usage. Now, what constitutes the data science process to unravel these behavioral patterns?
Data Collection:
Any insights gained through the data science process originate from the data itself; therefore, data collection is one of the most critical steps in the data science workflow. We need data that is not only relevant but also of high quality and available in large quantities. Using our example of understanding users' "liking" preferences on Instagram, essential data includes information on when a user clicks the “like” button, what types of content the user "liked", what the user did on the platform before and after clicking the “like” button, along with demographic details about the users.
We also need high-quality data because a dataset with a lot of missing values or errors, can lead to inaccurate insights! In addition, having large quantities of data is essential to ensure our sample is representative of the population. If our sample size is limited or biased towards specific categories, the results may be skewed. Imagine if our user data is solely from the USA; the “liking” behavior of USA users might significantly differ from that in Taiwan. Applying insights derived from USA users to the Taiwanese user base may yield ineffective results. This highlights a fundamental statistical concept: we draw a sample from the population and utilize it to comprehend patterns that can be generalized to the broader user base in the population.
Data Processing:
Data processing involves converting raw data into a format suitable for analysis. Critically, improper data processing can distort and alter the data, potentially giving rise to misleading results. Therefore, it's essential to utilize data processing methods that effectively highlight the information you wish to study.
For example, the timestamps capturing moments of clicking the “like” button may not be directly informative due to users being in various time zones globally. To standardize this, we might consider transforming the timestamps into labels like morning, afternoon, evening, and night given the respective time zones of the users.
Moreover, some rare events globally may introduce spikes of likes that do not represent typical user behavior (e.g., Taylor Swift unexpectedly announcing the release of a new song on Instagram). It is crucial to carefully evaluate whether including these anomalous “liking” behaviors will be beneficial for subsequent data analysis steps.
Additionally, corrections may be needed for data from users who accidentally “liked” content and later removed the “likes”. Mistakes in the data pipelines, such as recording "sharing" as "liking", should also be identified and rectified during this data cleaning step.
Overall, data processing ensures our sample accurately reflects characteristics and behaviors of the population by refining data to eliminate outliers and irrelevant information.
Data Analysis:
Data analysis usually starts with exploration. Exploratory data analysis (EDA) is to gain an understanding of the data quality, quantity, and characteristics. This process enables us to gather fundamental information within the data and uncover crucial trends and patterns. Sometimes, the insights gained from EDA are sufficient to inform decisions. Additionally, results from EDA can guide subsequent steps and more in-depth analyses.
Using our Instagram example, we might be interested in understanding the number of active users in the dataset and, on average, how many “likes” they clicked within a specific period. Further exploration could involve examining how these metrics are distributed based on user characteristics such as country of residence, age, and time of day. Additionally, we may want to delve into analyzing the number of “likes” per topic or the number of “likes” per order of posts on the feed. Simple statistical analyses at this stage can aid in determining whether the topic or the order of the posts provides more significance in users’ usage of the “like” button.
After completing exploratory data analysis, the subsequent step involves applying various advanced statistical and machine learning methods to gain deeper insights, predict outcomes, and prescribe the best course of action. Returning to our example, based on our exploratory analysis, we might decide to construct a model to predict, for each post, the probability of a user clicking the “like” button. The model can learn from characteristics of the posts such as the content topic, the post's order on the user feed, users’ age, and time of the day to identify patterns of users’ “liking” behavior. Successfully building a model to predict the likelihood of a post receiving “likes” allows us to strategically promote posts with a high probability of engagement at the optimal time and position on the users’ feed.
Data Visualization and Interpretation:
After all the data science effort, it is essential to consolidate all the discovered insights to construct a coherent data narrative or a "data product". With this data product, we can inform coworkers and stakeholders of any unexpected discoveries, share predictions made, provide suggestions that can aid in the decision-making process, and contribute to advancing our knowledge of the world. This consolidated presentation ensures that the valuable insights derived from the data analysis are effectively communicated and utilized for informed decision-making and continuous improvement.
Often, we convey these findings visually with graphs or charts that not only facilitate storytelling but also assist in decision-making processes. The objective is to craft a compelling story that effectively addresses the initial business or research questions. It is important that data visualization maintains transparency, avoiding any misleading representations and ensuring the delivery of a clear and informative message.
Using our example, our analysis may reveal a general tendency for different demographics of users to use the “like” buttons at different times of the day, and there may be a general inclination for users to “like” the first few posts they see on their feed, regardless of the content. Additionally, our model predicts the most possible posts for each user that they will “like”. Combining the general trend and the modeling results, we may be more confident in increasing overall Instagram engagement by promoting posts with a high probability of receiving “likes” from users.
Conclusion
Overall, data science serves as the catalyst for informed decision-making across various industries, providing a solid foundation for predictions and strategies based on empirical evidence rather than intuition. Next time when you are scrolling through your social media feed, consider the intricate process that occurs behind the scenes to tailor your experience. The order of posts, the appearance of specific ads, and the emergence of trending content are not arbitrary occurrences but the result of meticulous data science methodologies!
Reference
✨Further Reading:If you would like to read the Chinese version, please refer to《大數據背後的祕密——資料科學》
❤️Editor's Note
I am delighted to invite Dr. Wang Shaofang, a data scientist, to join the ranks of "CASE Scientific Reports"! Dr. Wang, who works in the United States, not only serves as a data scientist but has also devoted nine years to research in cognitive neuroscience. In this edition, Dr. Wang will use two articles to introduce the prominent field of our internet age - what exactly is "data science"? How is it researched? And how is it used? What's special is that both articles will be available in both Chinese and English versions simultaneously. If you're interested in practicing reading bilingual articles, please feel free to take a look! It will definitely be a rewarding experience for you!